Package: wsrf 1.7.30
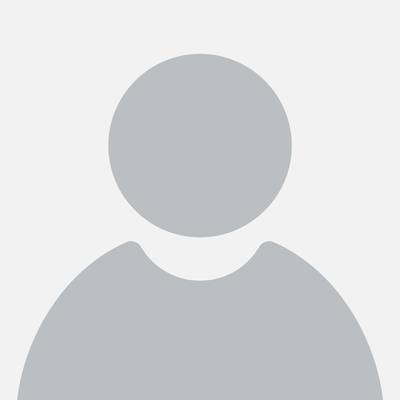
He Zhao
wsrf: Weighted Subspace Random Forest for Classification
A parallel implementation of Weighted Subspace Random Forest. The Weighted Subspace Random Forest algorithm was proposed in the International Journal of Data Warehousing and Mining by Baoxun Xu, Joshua Zhexue Huang, Graham Williams, Qiang Wang, and Yunming Ye (2012) <doi:10.4018/jdwm.2012040103>. The algorithm can classify very high-dimensional data with random forests built using small subspaces. A novel variable weighting method is used for variable subspace selection in place of the traditional random variable sampling.This new approach is particularly useful in building models from high-dimensional data.
Authors:
wsrf_1.7.30.tar.gz
wsrf_1.7.30.zip(r-4.5)wsrf_1.7.30.zip(r-4.4)wsrf_1.7.30.zip(r-4.3)
wsrf_1.7.30.tgz(r-4.5-x86_64)wsrf_1.7.30.tgz(r-4.5-arm64)wsrf_1.7.30.tgz(r-4.4-x86_64)wsrf_1.7.30.tgz(r-4.4-arm64)wsrf_1.7.30.tgz(r-4.3-x86_64)wsrf_1.7.30.tgz(r-4.3-arm64)
wsrf_1.7.30.tar.gz(r-4.5-noble)wsrf_1.7.30.tar.gz(r-4.4-noble)
wsrf.pdf |wsrf.html✨
wsrf/json (API)
NEWS
# Install 'wsrf' in R: |
install.packages('wsrf', repos = c('https://simonyansenzhao.r-universe.dev', 'https://cloud.r-project.org')) |
Bug tracker:https://github.com/simonyansenzhao/wsrf/issues
Last updated 2 years agofrom:f0f9e7c3de. Checks:1 OK, 7 NOTE, 3 WARNING. Indexed: yes.
Target | Result | Latest binary |
---|---|---|
Doc / Vignettes | OK | Feb 05 2025 |
R-4.5-win-x86_64 | WARNING | Feb 05 2025 |
R-4.5-mac-x86_64 | NOTE | Feb 05 2025 |
R-4.5-mac-aarch64 | NOTE | Feb 05 2025 |
R-4.5-linux-x86_64 | NOTE | Feb 05 2025 |
R-4.4-win-x86_64 | WARNING | Feb 05 2025 |
R-4.4-mac-x86_64 | NOTE | Feb 05 2025 |
R-4.4-mac-aarch64 | NOTE | Feb 05 2025 |
R-4.3-win-x86_64 | WARNING | Feb 05 2025 |
R-4.3-mac-x86_64 | NOTE | Feb 05 2025 |
R-4.3-mac-aarch64 | NOTE | Feb 05 2025 |
Exports:combinecombine.wsrfcorrelationcorrelation.wsrfimportanceoob.error.ratestrengthsubset.wsrfvarCounts.wsrfwsrf
Dependencies:Rcpp
Readme and manuals
Help Manual
Help page | Topics |
---|---|
Combine Ensembles of Trees | combine combine.wsrf |
Correlation | correlation correlation.wsrf |
Extract Variable Importance Measure | importance importance.wsrf |
Out-of-Bag Error Rate | oob.error.rate oob.error.rate.wsrf |
Predict Method for 'wsrf' Model | predict predict.wsrf |
Print Method for 'wsrf' Model | print print.wsrf |
Strength | strength strength.wsrf |
Subset of a Forest | subset subset.wsrf |
Number of Times of Variables Selected as Split Condition | varCounts.wsrf |
Build a Forest of Weighted Subspace Decision Trees | wsrf wsrf.default wsrf.formula |